Supercharging R&D in Life Sciences

Imagine a biotech company successfully integrating AI into its research and development (R&D) processes. Using AI algorithms, users in every division of the company can perform advanced analytics, predictive modeling and simulation studies. These capabilities allow them to quickly identify therapeutic targets, design more efficient clinical trials and enhance drug development. As a result, the company reduces costs, improves patient outcomes and boosts revenue. Additionally, it stays ahead of competitors by quickly adapting to new scientific discoveries and market demands.
How can your life sciences company achieve this level of success with AI? It all begins with a modern data strategy. Such a strategy will help you securely centralize, govern, share and collaborate on sensitive data, providing the foundation needed to power AI algorithms successfully.
Boosting drug discovery and development
Companies can save untold amounts of time and money by using AI to sift through vast stores of real-world data, omics data and clinical trial data. With this data, there are countless possibilities for what researchers can do. Here are some highlights:
Drug discovery models: Researchers can use AI models to predict protein structures, chemical properties and molecular docking, making the design and testing of new drug candidates much more efficient.
Synthetic data generation for secondary analysis: AI-generated data can mimic real-world data for secondary analysis, helping researchers validate their findings without compromising patient privacy.
Clinical text summarization: With natural language processing, AI can quickly summarize large volumes of clinical data, pulling out the important bits for researchers in no time.
Critical inquiries before prescribing AI
For these reasons and more, the industry is excited about AI. One report says using AI in drug discovery can slash the time and cost to bring a new drug to market by up to 75%. But on the flip side, it has been estimated that at least 80% of AI projects will fail. Reasons for this include poor data quality, inadequate risk controls, escalating costs and unclear business value.
To succeed in adopting AI, organizations need a robust data strategy that ensures that the data used to train AI models is high quality, relevant and accessible. Here are a few important questions companies should ask before they adopt AI:
How confident are we in the quality and governance of our data foundation?
How does this AI solution align with our overall data and business strategy and goals?
Have we clearly defined our KPIs? How often will we measure them to ensure ROI?
How quickly can we reasonably expect to see tangible results and value from this investment?
What is the total cost of ownership (TCO) for the solution or project, including additional costs like maintenance, training and future upgrades?
Can the solution evolve with our business needs and as technology and the industry continue to mature?
The importance of a modern data strategy
The answers to these questions will clearly demonstrate that AI is driving a major shift toward cloud technology. However, adopting AI can present significant challenges related to cost, complexity, security and governance. Retaining specialized hardware and software, along with skilled personnel, can strain budgets, while integrating AI with existing systems and managing large volumes of data can pose difficulties. Additionally, ensuring robust security and governance controls to protect sensitive data and comply with regulatory standards is a necessary but resource-consuming task.
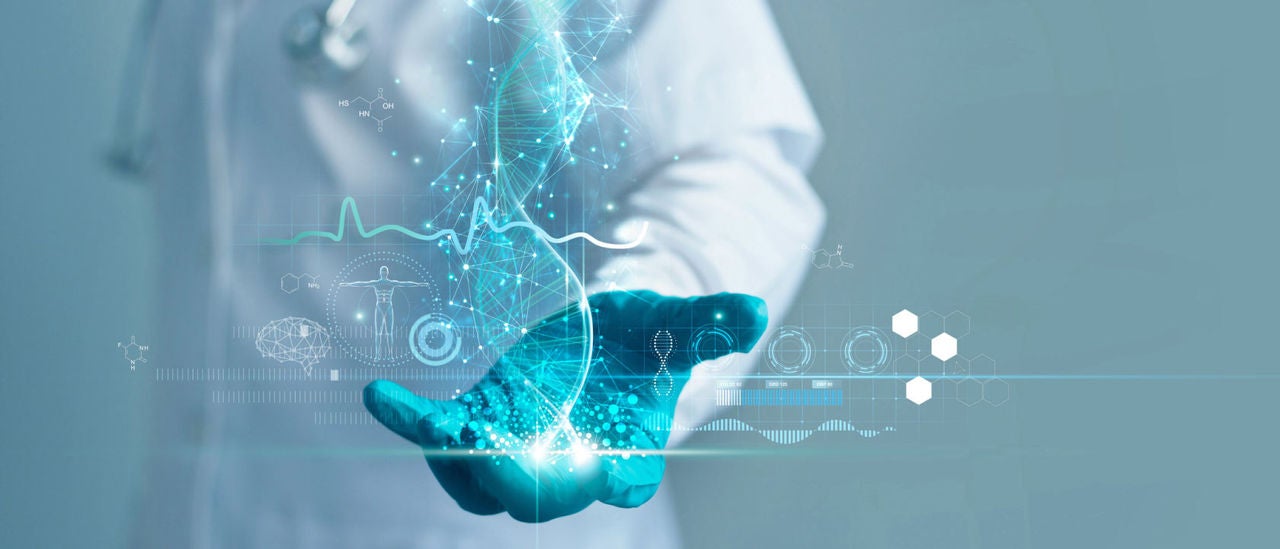
With a scalable, cloud-native platform, (companies) can optimize costs, simplify integration and enhance security, paving the way for successful AI adoption.
The good news is that companies with a modern data strategy can overcome these obstacles. With a scalable, cloud-native platform, they can optimize costs, simplify integration and enhance security, paving the way for successful AI adoption.
Here’s what else life sciences organizations can achieve by modernizing their infrastructure:
Data collaboration and operational efficiency
Improved data sharing and collaboration: Modern platforms integrate various data sources, allowing life sciences organizations to collect and share multimodal health data, such as clinical, genomic and imaging data, into a single, governed repository. This accelerates and simplifies data analysis.
Simplified operations: Legacy infrastructure often lacks interoperability, complicating and slowing drug development. Modern, fully managed data platforms streamline operations by seamlessly integrating various systems and data sources, accelerating drug development and optimizing R&D processes.
Increased scalability: On-premises systems are costly and cumbersome to scale for today’s massive data volumes and computing needs. Modern data platforms flexibly scale to support diverse data volumes and computational research workloads in parallel, avoiding resource competition and delays.
Third-party data access: Modern data platforms offer easy, secure access to valuable third-party data and apps, augmenting life sciences processes with enhanced insights. This integration also supports capabilities such as predictive analytics and advanced modeling.
Reduced costs and enhanced security
Lower TCO: Fully managed platforms handle maintenance and updates, freeing technical teams to focus on strategic initiatives. Pay-as-you-go pricing and scalable cloud platforms help optimize budget allocation and manage costs as data needs grow.
Enhanced privacy and security: Outdated systems may not support modern security protocols or meet regulatory standards on patient data, potentially leaving companies vulnerable to data breaches and noncompliance penalties. Modern data platforms include comprehensive and configurable data governance, enabling high levels of security and compliance.
To learn more about employing a modern data strategy that sets your life sciences research organization up for success, join us on Oct. 30 for our Accelerating Research and Clinical Development In Industry and Academia webinar. You can also download our ebook, 4 Ways Life Sciences Organizations Accelerate Their Development Pipelines with the AI Data Cloud.