Prioritization: The Pivot Point from POC to Production
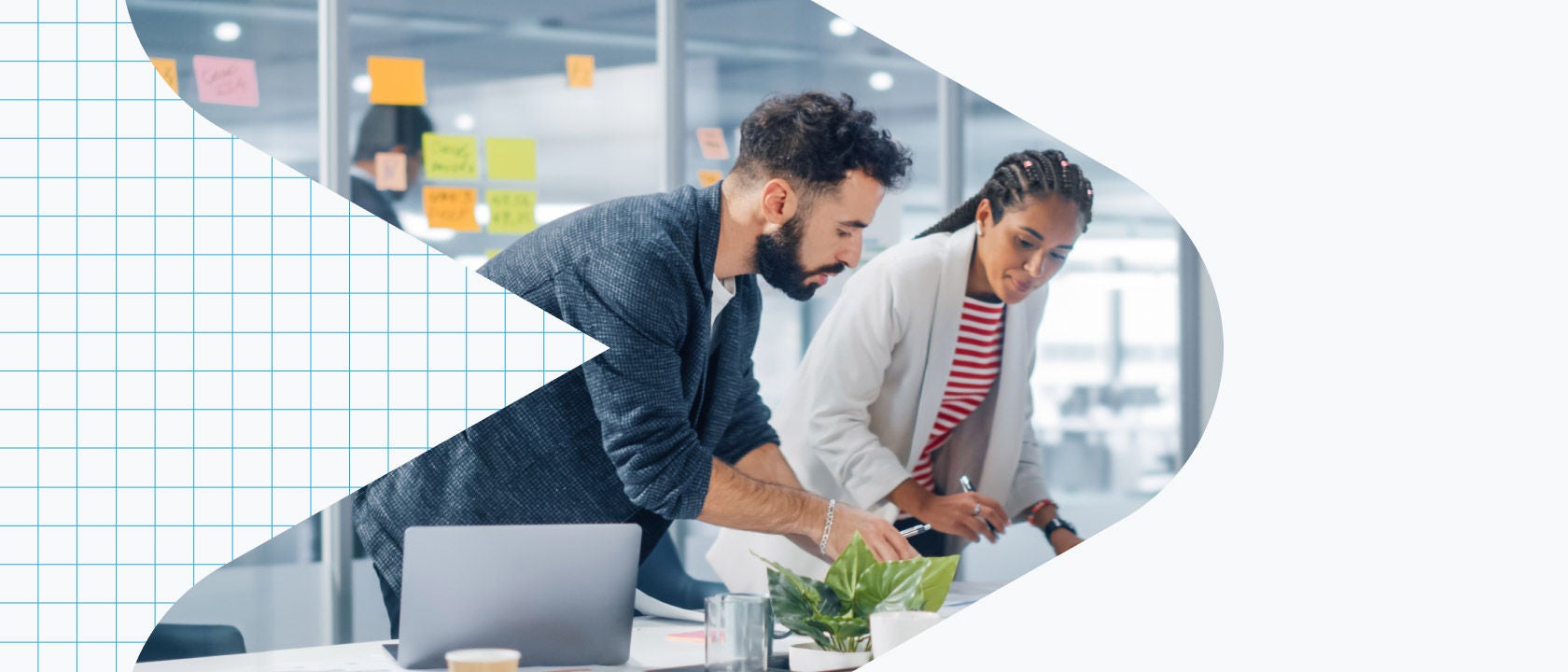
We often hear from customers that they’re excited about what they could do with data and AI but are not sure how to do it. Or that the tech teams are “all in” but they can’t convince the powers that be to move forward. It’s not that they don’t know what to do — they could list a number of initiatives or use cases that would benefit from insights from their data or to which they could apply AI. But many organizations seem to suffer from institutional paralysis.
At Snowflake Summit this summer, an executive from a major manufacturing company reflected wistfully, “If we only knew what we knew.” In other words, imagine all the things we could do if we could derive insights from all the data we have collected, all that we know about our customers or our products.
However, the challenge is knowing where to start. And, the answer lies in the prework: It’s what you do before you even fire up the tool. Imagine building a house without the plans. But even before the architect puts pencil to paper, there is a wish list of features for the dream house. How do you envision your life in the house? “Well, we’d like to take advantage of the gorgeous view the property has. To maximize the view, we’d like large picture windows or a wraparound porch. We’d also like to ensure the livability of the house with plenty of storage, so don’t forget the closet space.” Having recently gone through this process, I know it well. My wish list was long.
Then the hard part comes. You’ve got a budget and likely a set of rules to follow, such as the building code or the neighborhood ordinances. Constraints require making hard choices and prioritizing certain features over others. Ideally, you can start with a solid foundation and key features and allow for some of the others to be added in later. And maybe you’ll have to learn how to do some things yourself so that your desired features become DIY projects. But likely some of these projects will not see the light of day.
Sound familiar? Maybe you’ve built a house. Or maybe you’ve built a data and AI strategy and started to use it. You’ve evangelized the opportunities and experimented with a number of pilots, but now it’s time to determine what makes the cut.
The pivot point from plan to production: Prioritization
A recent Harvard Business Review article reports that 80% of AI projects don’t make it into production. That figure has been used to illustrate failure. However, a closer look reveals that some of those 80% were sidelined intentionally. The first step in moving from experimentation to implementation is selecting which projects or products should move forward. As one CDO described it to me years ago, the goal of prioritization is to ensure the view is worth the climb.
Ideas come from all parts of the business, and that’s a good thing. Diversity of ideas should be encouraged. AI sandboxes and hackathons encourage experimentation. Ultimately, however, those ideas must be put to the test. A rigorous and transparent prioritization framework helps ensure that proposed projects and products align with business goals and that it’s realistic for the team to create them.
The goal of prioritization is to ensure that the view is worth the climb.

The view. In the matrix above, the y-axis shows alignment with strategic business goals, broadly speaking. This is where you’d estimate the potential “view.”
Is the initiative aligned to business goals? Priority must be given to projects or products that have a direct link to specific business goals. Is there a business sponsor for the initiative? Data products won’t deliver value unless they drive action — that is, unless end users adopt them. This requires collaboration between business and data teams, and education on both sides about what is required and what is possible.
Can multiple business units benefit? Many data teams emphasize reuse as a requirement for prioritization: Value increases as more business units use the AI model or data product. For example, imagine that an electronics manufacturer wants to understand how its devices are used and by whom. A "product usage” data product could provide a customer 360 to see which products a customer was using and a product 360 to see which customers were using a particular product.
What is the anticipated return? This starts with identifying the metrics to be measured, ideally in terms of business value, and putting an estimated stake in the ground. Prioritization requires comparison across competing initiatives in concrete terms.
The climb. The x-axis reflects the complexity and feasibility of a specific initiative to determine whether it is achievable in terms of resources and risks. This is where you’d estimate the required “climb.”
Is the data available? The most critical requirement is the data to train the model. Is internal data easily available and accessible? Does the model require transformation or access to unstructured data? Would adequate training require external data, such as partner data or other third-party data, to mitigate risks of bias or hallucination?
What skills and tools are required? Here is where you have to be realistic about whether you have the resources to deliver, and what it takes to get there. An AI initiative shouldn’t feel like a sci-fi movie.
Are there risks involved or other concerns? For starters, the EU AI Act takes a risk-based approach to regulation and classifies AI systems into four different risk levels: unacceptable, high, limited and minimal risk. A number of tools can help assess risk level and provide guidance on the relevant regulations and requirements.
What is the cost to build and deploy? Ultimately, this is a business case and requires an estimation of the costs to build, deploy and maintain the AI model over time. These estimates should also include technology and data acquisition as well as training required. It’s not just about building an application.
The end result is a matrix in which each potential product or project can be placed and evaluated in terms of the predicted view and the required climb.
The two-by-two matrix yields the following categories:
High-value quick wins: Great view, short and easy climb. These are the initiatives that fully align with strategic business objectives and are considered either less complex or highly feasible.
Don’t bother: Limited view, tough slog. These are considered harder and lower-potential-value initiatives that are not necessarily worth the effort.
Long-term investments: Great view, but long and steep climb.These initiatives are expected to deliver significant value, but they are considered more complex or come with significantly more risk or resource requirements. These might be broken down into smaller initiatives or component data products that could later be aggregated to deliver the full value.
Investigate further: Limited view, but relatively short and easy climb. These initiatives are expected to deliver some value (or they wouldn’t be proposed) but are less strategically aligned. However, they are considered relatively easy to deliver. It’s more of a “could do” but only with remaining time or resources, hence the lower priority.
This isn’t an optional process. The move from proof of concept to product/project involves hard choices. A formal prioritization framework ensures that initiatives are evaluated equally and transparently, that initiatives support business strategy, that initiatives are feasible for the organization and that resource requirements and expected outcomes align.
To hear more about how Snowflake customers have traveled the AI journey from evangelism and experimentation to operationalization and transformation, check out “The Data Executive’s Guide to Effective AI.”